By Carlos Ponce
In this study, we pursue a long-standing question in neuroscience: how does the brain represent the visual world? When our eyes receive images of natural scenes, neurons in the early visual system cannot transmit this information to the rest of the brain by copying these images “pixel by pixel.” Instead, we know the brain must compress these scenes into a simpler scheme that preserves information about some visual features, and throws away others. These features must be simpler than the original scenes, but how much simpler? So far, there has been no clear way to approach this question because answers required a way to “translate” information in action potentials back into natural images, using the activity of neurons deep in visual cortex, many synapses away from the eye. While this was implausible for a long time, the emergence of new computational models has provided some new opportunities. We used generative adversarial networks (or image generators), which learn to represent and generate naturalistic images. The image generators worked under the guidance of cortical neurons in monkey visual cortex, creating synthetic images that represented the visual features encoded by the same neurons. This is a method invented here at Harvard with the Livingstone and Kreiman laboratories (Ponce, Xiao et al., 2019, Cell). In this new paper, we extracted synthetic images from many different brain regions, and used information-theoretic measures to describe the complexity of these synthetic images — how simple they are relative to natural scenes. We show how their stored information can be used to solve different decoding problems of vision, and further establish that these representations can be related to spontaneous viewing behaviors shown by the monkeys — a key prediction from theories of efficient coding in the brain.
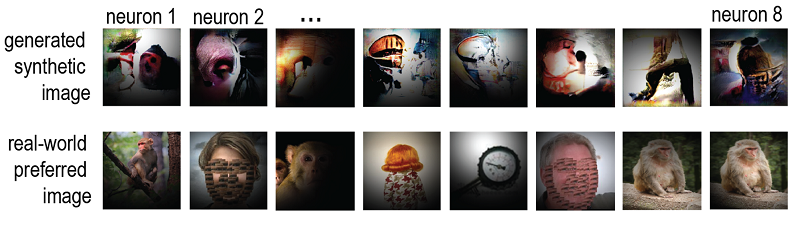
Synthetic images. Each column shows the computer-generated image that emerged from the interaction of one neuron and the machine learning generator (top), and an example of a real-world image that also elicits strong responses from the same neuron (bottom).
Carlos R. Ponce is an Assistant Professor in the Department of Neurobiology at Harvard Medical School.
This story will also be featured in the Department of Neurobiology’s newsletter, ‘The Action Potential’.
Learn more in the original research article:
Visual prototypes in the ventral stream are attuned to complexity and gaze behavior. Rose O, Johnson J, Wang B, Ponce CR. Nat Commun. 2021 Nov 18;12(1):6723. doi: 10.1038/s41467-021-27027-8. PMID: 34795262; PMCID: PMC8602238.
News Types: Community Stories