By Jacob Prince, George Alvarez, and Talia Konkle
Our brains have an impressive ability to instantly recognize faces, places, words, and objects, thanks to different regions in visual cortex that respond strongly to these categories. But why and how do these category-selective areas develop? Are they built-in brain “modules”, each dedicated to recognizing a specific category, or is it a more general system that learns complex patterns from the world around us, without any category-specific rules? Decades after scientists first identified category-selective regions with brain imaging, these puzzles have remained unsolved.
To investigate these questions, our team used cutting-edge AI models that function like a simulated set of brain circuits, processing images and telling us what’s in them, just as our own visual systems do. We employed a form of machine learning called self-supervised contrastive learning. In this approach, instead of explicitly labeling images (such as identifying an image as a “face” or “object”), we trained the model to distinguish one image from all others based on visual differences alone. Over time, the model naturally began to group similar images together, forming clusters that corresponded to hundreds of distinct visual entities and objects. This structure emerged solely from the model’s exposure to vast amounts of natural images—without any dedicated parts for processing specific items like faces or bodies or words, and without any explicit knowledge of what a visual “category” even is.
This learning framework, which we call contrastive coding, suggests a way our own brains might develop visual categories: by mere exposure to a rich variety of visual experiences. This means we may not need built-in, category-specific “modules” in the brain; instead, our visual systems can learn to recognize and separate out important categories just by interacting with the visual world around us.
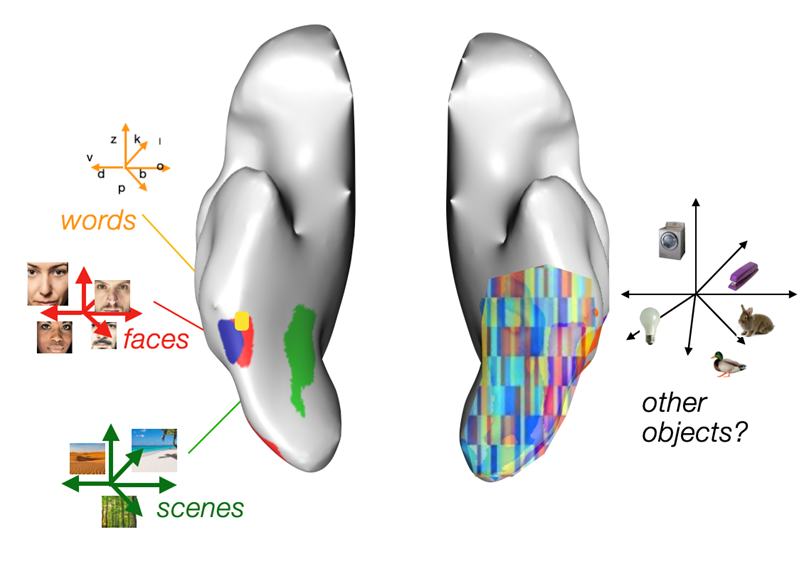
The ventral visual stream contains regions selective for categories like faces, scenes, and words, which have been traditionally viewed as independent brain “modules”. A competing view suggests that these inputs are instead represented through distributed activity patterns across neural populations, involving many different regions. New research using AI models and neuroimaging data provides a way to resolve this longstanding debate in visual neuroscience
This model provided us a new path to understanding how category-selective brain regions emerge and function in the context of object recognition. As the model processed more images, it formed distinct groups of selective units that responded strongly to certain categories, similar to how the brain has regions like the fusiform face frea (FFA) for faces or the visual word form area (VWFA) for words. We also ran tests on the model to see what would happen if we “silenced” some of these category-selective groups. Disrupting, say, the face-selective units in the model reduced its ability to recognize categories that rely on facial features, echoing what we see in humans with brain injuries to specific visual areas. These disruptions also revealed the model’s reliance on certain units to recognize specific categories, highlighting that some parts of our visual cortex may indeed play a “privileged” role in processing certain kinds of information.
Finally, we wanted to see if our model’s visual processing was similar to that of the human brain. We used high-quality brain imaging data showing how people’s brains respond when looking at thousands of natural scenes and compared it to our model’s predictions. Remarkably, the model’s category-selective units aligned closely with actual brain activity, especially in areas responsible for recognizing faces and scenes. This alignment strengthens the idea that contrastive learning captures fundamental ways our brains separate out categories, organizing visual information naturally without needing hard-coded mechanisms for each specific category.
Our findings reveal that the visual system may not need pre-set, category-specific rules to “see” the world in categories. Instead, it can learn to separate faces, bodies, objects, and more, simply by learning to recognize and distinguish a variety of different views of the world from each other. This work provides new insight into how our brains might naturally develop the ability to recognize visual categories through everyday experiences as we grow and learn.
Jacob Prince is a 4th-year PhD candidate in the Vision Sciences Lab, Department of Psychology, working with Professors Talia Konkle and George Alvarez.
Talia Konkle is a professor of psychology at Harvard University.
George Alvarez is the Fred Kavli Professor of Neuroscience at Harvard University.
Learn more in the original research article:
Prince JS, Alvarez GA, Konkle T. Contrastive learning explains the emergence and function of visual category-selective regions. Sci Adv. 2024 Sep 27;10(39):eadl1776. doi: 10.1126/sciadv.adl1776. Epub 2024 Sep 25. PMID: 39321304; PMCID: PMC11423896.
News Types: Community Stories