By Davide Valeriani
Imagine waking up one day and noticing your voice has frequent breaks. Alarmed, you go see your primary care physician, who refers you to an otolaryngologist. After observing your vocal folds with a laryngoscopic exam, the doctor suspects muscle tension dysphonia, and refers you to a speech language pathologist (SLP). More time passes and you the get your appointment with the SLP, who suspects essential voice tremor, and refers you to a neurologist to exclude other pathologies. After several weeks, the neurologist visits you and orders a magnetic resonance imaging (MRI) exam, which takes a few days to be scheduled. After doing the MRI, you wait for the follow-up visit, and the neurologist confirms the absence of neurological disorders. Confused, you then decide to see another otolaryngologist, who has extensive experience in dystonia. This doctor sees your symptoms and decides to diagnose you with laryngeal dystonia. Four years have passed since your first symptoms.
This exemplary journey represents the struggle of thousands of patients with different forms of dystonia to receive an accurate diagnosis. Dystonia is a rare neurological movement disorder characterized by involuntary muscle contractions, leading to abnormal movements and postures. Its pathophysiology is unknown and there is no biomarker for dystonia, which lead to significant delays in the diagnosis, extending up to 10 years after the first symptoms. Clinicians often disagree with each other about the diagnosis of a dystonia patient (as we have seen above), particularly if they are not specialized in movement disorders.
Several neuroimaging studies have shown widespread alterations of brain microstructure and function in patients with this disorder. So, we thought these alterations could serve as a biomarker to diagnose dystonia from MRI data.
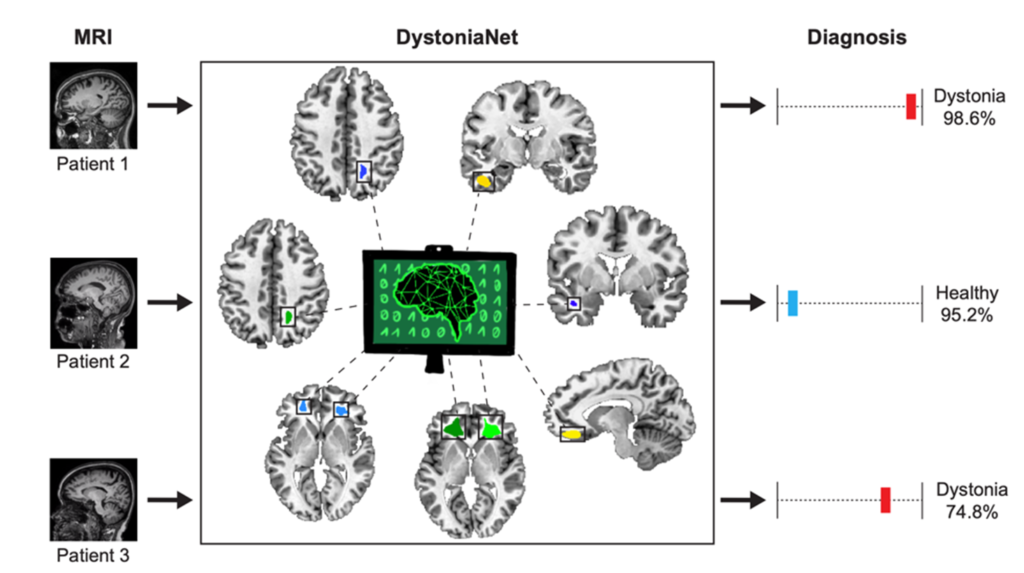
DystoniaNet receives a raw MRI as input, and uses its data-driven biomarker to make a probabilistic diagnosis of dystonia for each patient.
Leveraging on the advances of machine learning, we developed DystoniaNet, a 3D convolutional neural network capable of diagnosing dystonia from raw structural MRI data. We trained and optimized DystoniaNet on 440 patients and controls using a data-driven approach. We also provided DystoniaNet with the capability of estimating its uncertainty and referring difficult cases to further examinations. When testing DystoniaNet on an independent test set of 172 patients with three different forms of dystonia, it achieved an accuracy of 98.8% across all forms, referring only 3.5% of patients to further examination. We also verified its high specificity on 1,480 healthy controls, whose brain MRIs were gathered from publicly-available datasets. DystoniaNet correctly classified 96.9% of controls, referring 2.6% to further examination.
We also made DystoniaNet interpretable, by analyzing its internal model to uncover a novel data-driven biomarker of dystonia composed of corpus callosum, anterior and posterior thalamic radiations, inferior fronto-occipital fasciculus, and inferior temporal and superior orbital gyri. All these regions have been previously found microstructurally abnormal in patients with dystonia, but DystoniaNet did not know: it discovered them automatically from the data!
In addition of being extremely accurate, DystoniaNet is also very fast: it takes only a fraction of a second (0.36 s, on average) to make a diagnosis. We designed its architecture with the environmental efficiency in mind: DystoniaNet is up to six orders of magnitudes more energy efficient than other deep learning platforms, for example, the popular AlexNet.
We hope that DystoniaNet would transform the clinical management of dystonia by reducing the rate of mis- and underdiagnosis, and by speeding up the diagnostic process. We envision DystoniaNet to be implemented in the clinics as a cloud-based platform, where the clinician uploads the MRI of a patient and receives a diagnosis in seconds (see video below).
This project was also featured in HMS News.
Davide Valeriani is a postdoc in the Dystonia and Speech Motor Control Laboratory at the Massachusetts Eye and Ear Infirmary.
Learn more in the original research article:
Valeriani D, Simonyan K (2020). A microstructural neural network biomarker for dystonia diagnosis identified by a DystoniaNet deep learning platform. PNAS. doi:10.1073/pnas.2009165117
News Types: Community Stories