By Alex Bardon
Certain neurons in the visual system are known to respond more strongly to specific categories of objects. One type of object-selective neuron that has been of particular interest in visual neuroscience is face neurons. In both humans and monkeys, researchers have found examples of such neurons, which fire strongly when the subject is shown a picture of a face, and much less when the subject is shown a picture of other objects.
We explored whether these so-called face neurons truly respond categorically to faces and only faces, or whether they respond to visual features that are present in faces but do not necessarily count as a face. As an example of a categorical neuron, consider a hypothetical “tennis neuron.” It would fire strongly in response to both a tennis racket and a tennis ball, yet not respond to a lemon, which may look visually like a tennis ball but does not fall in the same category. Similarly, for a categorical face neuron we would expect that it fires strongly to all different types of faces, but does not respond to other objects that share visual features with but are not actually faces.
To distinguish between these two possibilities, we used an algorithm called XDream to generate stimuli that strongly drove face and non-face neuron responses. The XDream algorithm is based on a generative neural network and is, therefore, not limited to realistic images of objects. We then asked people to assess the “faceness” of these generated images and compared the human assessments with the neural responses to these images.
Face neurons responded to their generated images as strongly as they did to photos of faces (or in some cases even more strongly). Yet, people in our study did not perceive these images generated from face neurons to be actual faces. On the other hand, images generated from face neurons were generally rated as more face-like than images generated from non-face neurons. Furthermore, we found that among photos of objects, the response of face neurons was correlated with people’s ratings of how face-like the object was, even if the photos only contained non-face objects (for example, a jack-o-lantern was rated more face-like than a chest-of-drawers). However, this relationship did not hold for the generated images, which caused high face neuron firing but received low faceness ratings.
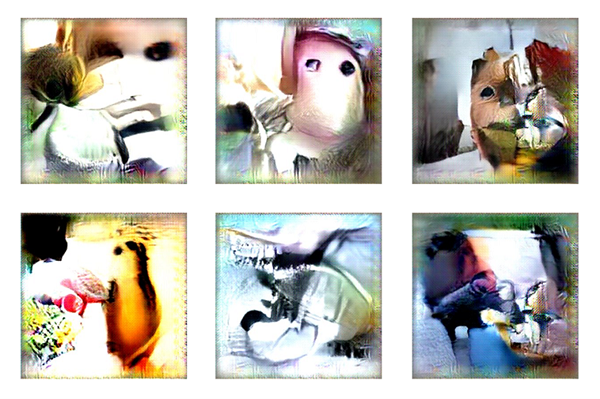
Top row: Images generated to strongly activate face neurons using the XDream algorithm. Bottom row: Images generated for non-face neurons.
Our results suggest that that so-called face neurons are better described as tuned to visual features rather than semantic categories. The results highlight the challenges associated with word models when thinking about visual neurons that appear to be category selective and emphasize the need for more quantitative theories of neuronal tuning in the visual cortex.
Alex Bardon just finished her undergrad at Caltech and will be starting her PhD in Brain and Cognitive Sciences at MIT in the fall. This work was done while she was an undergraduate research fellow in the Kreiman lab at Harvard.
Watch a video discussing this paper here.
Learn more in the original research article:
Face neurons encode nonsemantic features.
Bardon A, Xiao W, Ponce CR, Livingstone MS, Kreiman G. Proc Natl Acad Sci U S A. 2022 Apr 19;119(16):e2118705119.
News Types: Community Stories