By Anna Kutschireiter & Jan Drugowitsch
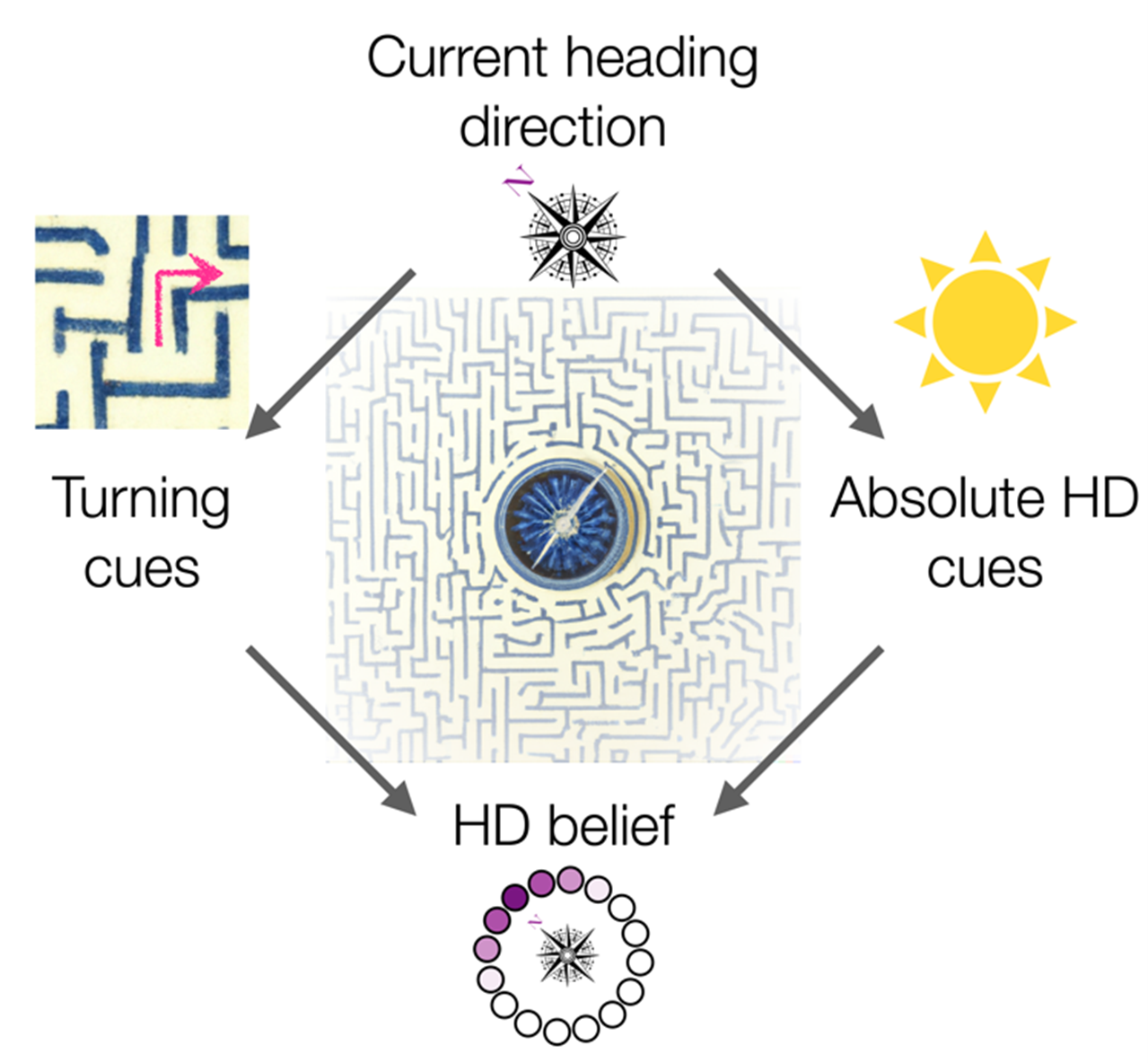
We update our cognitive variables, like heading direction (HD), based on different sensory cues, forming a “belief.” In ring attractors, this belief is represented as a bump of neural activity. In our model, the height of the bump scales with how certain we are about the belief.
Our ability to remember and process information is closely tied to uncertainties. For example, when navigating a maze, the uncertainty in our internal heading direction (HD) estimate increases with every turn we take. This increasing uncertainty is not a flaw, but a valuable feature. It allows us to accurately evaluate new sensory information, such as the position of the sun, even when it may be unreliable, and compare it with our own internal HD estimate. Popular “ring attractor” models of working memory models represent estimates like heading direction (HD) using a locally elevated “bump” of neural activity. By design, they lack a mechanism to incorporate uncertainty. We asked how these models need to be modified to include uncertainty in both the pattern of neural activity and the process of integrating new sensory evidence.
Surprisingly, our findings suggest that we don’t need to make extensive modifications to conventional ring attractors. They already possess the necessary characteristics if we associate the height of their activity bump with the estimate’s certainty. This further led us to explore the essential network patterns, or “motifs”, required in a simple recurrent neural network to update both the estimate and its associated uncertainty. To our surprise, conventional ring attractor models already exhibited all of these motifs, but need to be tuned to feature unconventionally slow internal dynamics.
While we can fine-tune the neural connection strengths in a ring attractor network to optimally make use of both its own uncertainty and the reliability of external cues, we also discovered that a wide range of connections can achieve near-optimal performance. To illustrate this, we more closely examined an example of a real biological ring attractor network: the Drosophila (fruit fly) HD system which is known to implement HD tracking in a network whose connectivity constraints are know from the Janelia Hemibrain database, and that is more distributed than the conventional ring attractor networks that we examined. Nonetheless, we could show analytically and in simulations that the fly’s network, despite its more constraint and complex structure can – at least in principle – support the tracking of uncertainty along with the HD estimate.
In summary, our work sheds light on how ring attractors can represent and update uncertainty alongside the actual estimate, which is a crucial aspect of neural computation
Anna Kutschireiter, PhD, is a former postdoc in Jan Drugowitsch’s lab. Jan Drugowitsch is a professor in the Department of Neurobiology.
Learn more in the original research article:
Kutschireiter A, Basnak MA, Wilson RI, Drugowitsch J. Bayesian inference in ring attractor networks. Proc Natl Acad Sci U S A. 2023 Feb 28;120(9):e2210622120. Epub 2023 Feb 22.
News Types: Community Stories